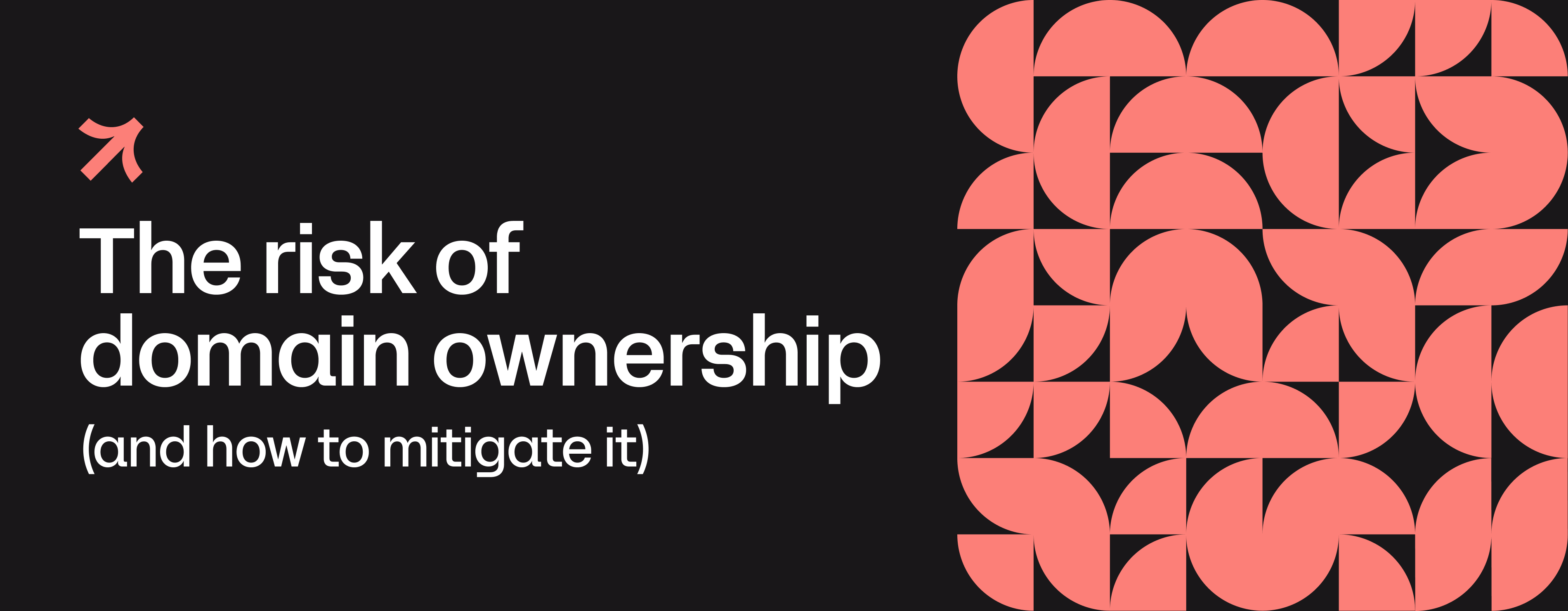
The risk of domain ownership (and how to mitigate it)
At a certain scale the org structure around data functions needs to be a hybrid of centralized and decentralized. We need a central data team to build and maintain the company’s data foundation. We need embedded data practitioners to become domain experts who can proactively provide domain-specific data strategy to the business.
This was one of the clearest take-aways from my guests in season 1 of The Right Track. (Listen directly to Erik Bernhardsson, Emilie Shario or Maura Church talking about this).
In my last post, I introduced the idea of the “data governance dilemma”, the challenges around getting good data fast, and our vision for a new way to tackle data governance at scale.
I also introduced my journey to accepting the data mesh principles as more than yet-another-framework-we-don’t-need. Because at their core, the data mesh principles are domain-oriented decentralized data ownership and architecture, data as a product, self-serve data infrastructure as a platform, and federated computational governance. Which is everything I subscribe to in building a data culture.
To continue this conversation, we need to align on what a domain is:
What is a “domain” in the data mesh principles?
A domain is a team dedicated to a certain function. It might be a team that works on a particular feature, like the “search” team. It could be the marketing team, or the finance team. They all have different needs from the data and they need to be able to influence what data they get access to.
At the core, we think of a “domain” when we refer to an area or problem space within a product or organization. Usually attached to a domain is a team that operates with some level of autonomy. It might have its own dedicated Product Manager, developers, and embedded analysts who specialize in that domain. They might also just be a group of analysts who report to the CFO.
It is likely responsible for making a plethora of decisions and design choices to evolve their feature or product area or internal data product offerings. And in order to be most effective in these decisions, they need reliable data.
It’s an all too common story that the domain team are “data consumers” who work with the data team to get the insights they need. But as we’ll explain,
data practitioners should think of domain owners less as people who receive data, but rather as colleagues who should design and create their own data. We call this paradigm shift a move towards “domain-driven ownership”.
In a data mesh framework, the domains (such as the checkout team, the onboarding team, finance, etc.) are responsible for their own data—from collecting to serving, and ideally in a way that the rest of the org can also consume it.
What does this mean for data governance? And specifically the governance of event based data.
Why domain ownership (centrally governed) is the only way to scale data
I’m just going to say it: You can’t successfully scale your data without optimizing for domain ownership. And you can’t optimize domain ownership without centralized governance. Without these things, you end up with unhappy embedded data practitioners, an unhappy central data team, crappy data, and slow moving teams.
Domain teams produce the most relevant data
The people closest to their domain area know it best, and are therefore best placed to take ownership of analytics for their space or product feature. As domain leaders are so familiar with their area, they automatically have an advantage in understanding and communicating its complexities.
When domain teams can create their own analytics and take care of their data needs themselves, they’re free from having to overcome communication barriers with a central team that may be less plugged into the minutiae of their specific area. This approach makes a lot of sense at the modeling level—the same principles also apply when it comes to data design.
It boils down to the fact that decisions around tracking for domains should be made at the local level, where local expertise is most prevalent, but within a framework that works across all domains. The further we get from domain expertise, the greater the hurdles of communication, understanding, and knowledge when it comes to designing and creating great data.
Domain teams will inevitably take control of their own data
Domains need data. If they can’t get it fast enough through the system you have, they will go rogue and run on their own. Maybe they’ll only go so far as to start sending the events they need into the analytics tools you already have. But we also see time and time again that they simply buy their own tool and start using that fully on their own. In other words:
Domains will inevitably generate the data they need. It's just a question of whether there’s a system in place that's well designed enough that they are willing to work within it.
They need to be able to get their data at the speed they prefer, but with the guardrails the central team prefers.
The central team wants to empower this, because otherwise they’re stuck solving data problems for the domains, and don’t have time to build the foundation.
On the other hand, it’s important for domains to be able to run fast without being blocked by a the central data team’s fifty-year long backlog. Let’s take a look at these advantages in more detail.
Clunky governance leads to domains going rogue
Data bottlenecks are the enemy of speed and data quality. When analytics changes are hindered by a review or approval process, it leads to data breadlines, where people line up for their analytics needs. Frustration builds and data doesn’t flow to the people when they need it. Most importantly:
Data governance bottlenecks lead to people bypassing the governing system because product managers or product engineers can’t afford to delay shipping while the data team catches up.
The solution is not to hire more people or to forgo data review — because that leads to bad data and a “wild west” where consistency in data design goes out the window. Instead, the solution is to provide the right frameworks and guardrails that automatically keep new data within the confines of predetermined data design rules, and let the domains run free and own their data within those confines.
As one Avo user put it, domain ownership means “analytics is lock-step with any new features and functions that we’re launching”. And speed of data delivery, particularly when you’re assessing first impressions of a new product release, can be a huge boon for analysts and product teams.
Automated guardrails remove the pain of manual review processes
Data design reviews, while necessary, don’t just slow things down. They’re often a painful experience for data teams as well. Whether it’s wading through a vast tracking spreadsheet or struggling to interpret events in a YAML repository, we’ve seen time and again how draining the review process can be.
The more we can empower domain leaders to design great data, the less friction we’ll see in our data workflows. By setting robust data design standards and building automatic guardrails (think linters or Grammarly for your data planning) and allowing domain teams to create great data themselves, we can reduce the need for input from central data teams. This frees up time, headspace, and eliminates frustration from both sides of the coin—both central data practitioners and embedded data domain experts.
The risk and mitigation: Let domains own their data (scary) and empower them with centralized governance and support
As someone responsible for the company wide data quality it’s scary to let domains own their own data. Because eventually you’re accountable for their work. You’re the one who the CEO comes to and asks why the retention dashboard isn’t working. And that sucks as a feeling. But the reality is:
We can’t scale data without letting domains own their own data, because the alternative is a bottleneck mayhem. And that will lead to domains going rogue anyway, which leads to data mayhem. So the only way to solve this is with domain-oriented ownership with centralized governance.
The greater autonomy and empowerment we can afford domain teams when it comes to data design, the better. It makes for a more efficient workflow with less friction, and it frees up crucial bandwidth for centralized data teams.
But simply unleashing domain experts to run with data isn’t the answer. They need the right mix of empowerment, support, and safety to create data that’s consistent with central standards.
In our next post, we’ll cover how this might be achieved. Namely: how might we provide the conditions for domain owners to succeed as autonomous data creators, while adhering to robust data quality standards. How can we design a system that let’s people design and implement data within a framework, without having to depend on them to read a manual? (Because who are we kidding, people do not read manuals).
Subscribe to get notified of the next post in this series on Central Governance with Domain Ownership: How to empower anyone to create great data.